All You Need to Know About Machine Learning
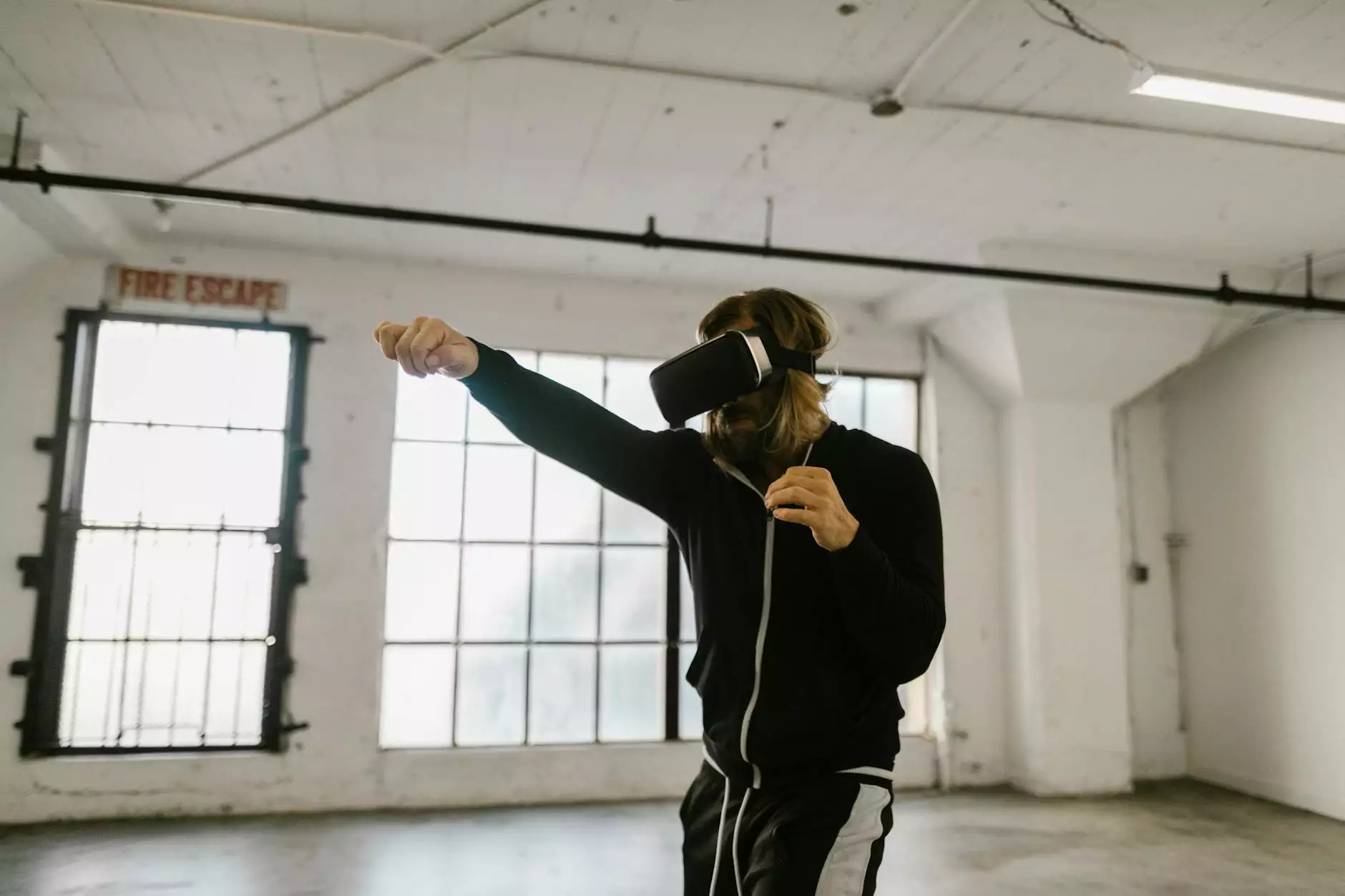
Machine Learning is a revolutionary technology that is transforming industries and reshaping the world as we know it. This guide will break down this complex field into easy-to-understand segments, covering its definitions, types, applications, benefits, and future trends. If you're keen to grasp the essentials of machine learning, you're in the right place!
What is Machine Learning?
At its core, machine learning is a subfield of artificial intelligence (AI). It involves the development of algorithms that allow computers to learn from and make predictions based on data. Rather than being explicitly programmed to perform a task, these algorithms use statistical techniques to improve their performance as they are exposed to more data.
Types of Machine Learning
Machine learning can be broadly categorized into three main types:
- Supervised Learning: This type of learning uses labeled data to train algorithms. The model learns from the input-output pairs and can predict outcomes for new, unseen data. Common applications include email filtering and credit scoring.
- Unsupervised Learning: In this type, the algorithm is trained on data that does not have labels. The objective here is to explore the data to find hidden patterns or intrinsic structures. Examples include clustering and association.
- Reinforcement Learning: This type of learning is modeled after behavioral psychology. Algorithms learn to make decisions by receiving rewards or penalties for their actions. It’s commonly used in robotics and gameplay.
Key Components of Machine Learning
To understand machine learning fully, it's essential to grasp its key components:
- Data: The foundation of machine learning. Quality data in sufficient quantity is key to training robust models.
- Algorithms: These are the mathematical functions that process the data and produce outputs. Different algorithms suit different tasks.
- Model: A representation of what the algorithm has learned from the data. The model can then be used to make predictions or decisions based on new data.
Applications of Machine Learning
Machine learning is utilized across various sectors, yielding significant improvements in efficiency and accuracy. Here are some notable applications:
Healthcare
In the healthcare industry, machine learning is revolutionizing patient care. Algorithms are used for:
- Predictive Analytics: Predicting patient outcomes and potential diseases based on historical data.
- Medical Imaging: Enhancing the accuracy of diagnostics through improved analysis of X-rays, MRIs, and CT scans.
- Personalized Medicine: Tailoring treatment plans based on individual patient data.
Finance
Machine learning plays a pivotal role in the finance sector with applications such as:
- Fraud Detection: Identifying unusual patterns and activities to prevent fraud.
- Algorithmic Trading: Using machine learning algorithms to automate trading strategies.
- Risk Management: Assessing and predicting financial risk through data analysis.
Retail
In retail, businesses leverage machine learning for:
- Customer Personalization: Analyzing consumer behavior to provide tailored product recommendations.
- Inventory Management: Predicting stock levels and optimizing supply chain operations.
- Sales Forecasting: Enhancing forecasting accuracy through historical sales data analysis.
Benefits of Machine Learning
Machine learning offers numerous advantages, enhancing business operations and decision-making. Some key benefits include:
- Increased Efficiency: Automating routine tasks allows organizations to focus on strategic activities.
- Improved Decision-Making: Data-driven insights lead to better business decisions.
- Cost Reduction: Streamlining operations and minimizing errors can result in significant cost savings.
- Enhanced Customer Experience: Personalized services improve customer satisfaction and loyalty.
Challenges in Machine Learning
Despite its advantages, machine learning is not without challenges. Understanding these can help businesses navigate potential pitfalls:
- Data Quality: Poor quality data can lead to inaccurate model predictions.
- Overfitting: This occurs when a model learns noise in the training data instead of the actual patterns, resulting in poor performance on new data.
- Resource Intensive: Training complex models can require significant computational resources and time.
- Ethical Concerns: Issues surrounding data privacy and algorithmic bias must be addressed to build trust.
The Future of Machine Learning
The future of machine learning is bright and filled with possibilities. As technology continues to advance, several trends are emerging:
- Integration with AI: Machine learning will increasingly be integrated into AI systems, enhancing their capabilities.
- Edge Computing: Performing data processing at the 'edge' will reduce latency and improve efficiency.
- Explainable AI: There will be a heightened focus on making machine learning models transparent and understandable.
- Federated Learning: This approach allows for collaborative model training while keeping data localized, enhancing privacy.
Conclusion
In conclusion, understanding machine learning is essential for anyone looking to remain relevant in today's data-driven world. From its basic concepts to its applications and future trends, this guide has provided a comprehensive overview of everything you need to know about machine learning. As industries continue to embrace this technology, staying informed will enable you to leverage its potential for enhanced productivity and innovation.
all you need to know about machine learning